How modern is the MoMA?
Data analysis and visualizationAs my capstone project for Parsons’ data visualization certificate, I investigated how the Museum of Modern Art’s collection grew over time.
Data cleansing, analysis, and visualization done with Tableau. Visual design in Sketch.
View the full project here.
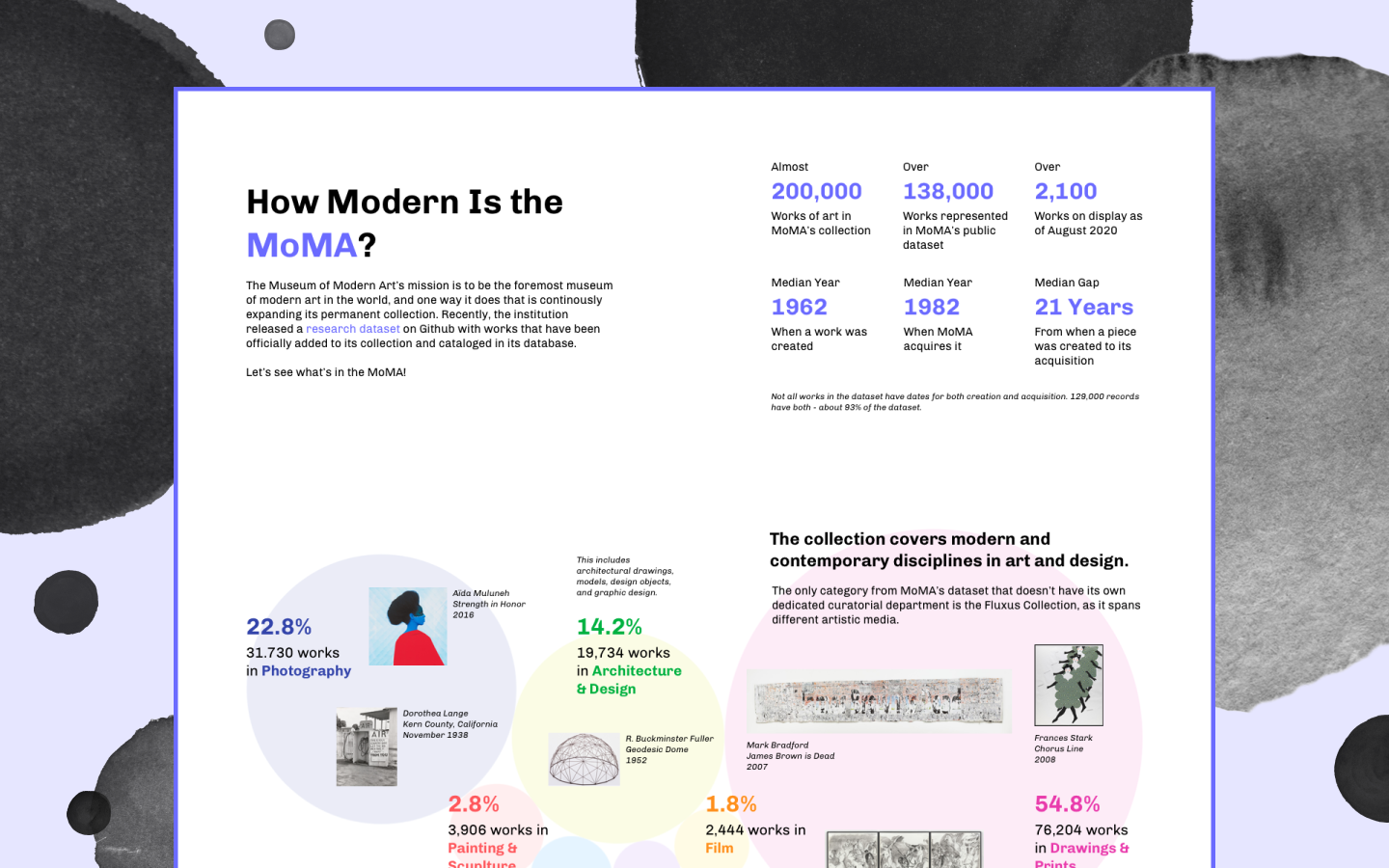
Going in questions first
At the time of this project, MoMA’s public dataset on Github contained ~139k works, out of ~200k in the full collection. It has basic metadata for each work, including title, artist, date made, medium, dimensions, and date acquired.While digging through the data, I wanted to ask how modern is the MoMA?
The fact that there were 2 time series caught my eye.
- When art was created wasn’t straightforward. Instead of dates, this column was free text where anything goes. It could be date ranges, estimates, or a paragraph of context.
- When MoMA acquired art was comprehensive and cleanly formatted. Not only were there dates, but I could see sources/methods: purchased, donated, gifted by the artist, etc.
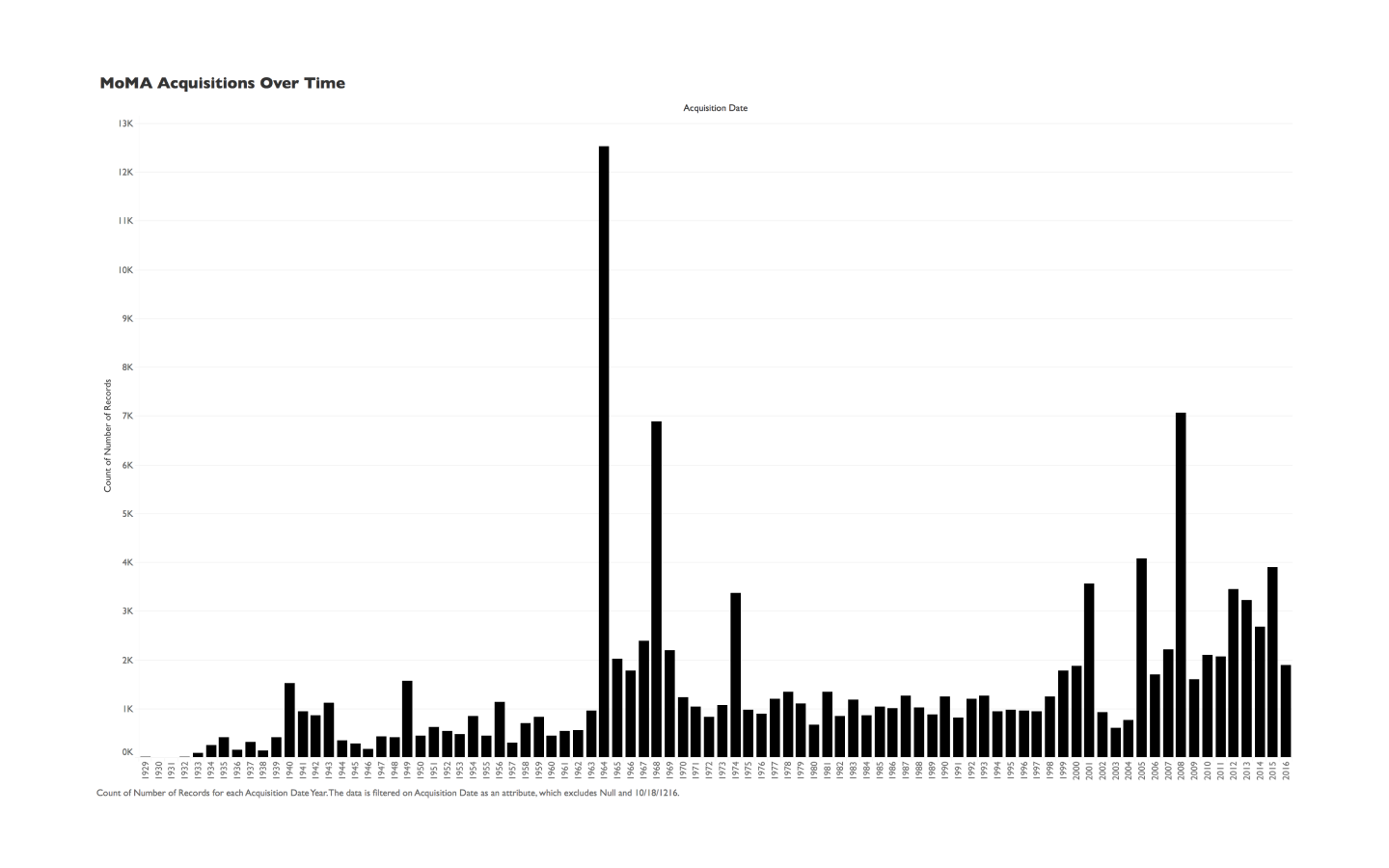

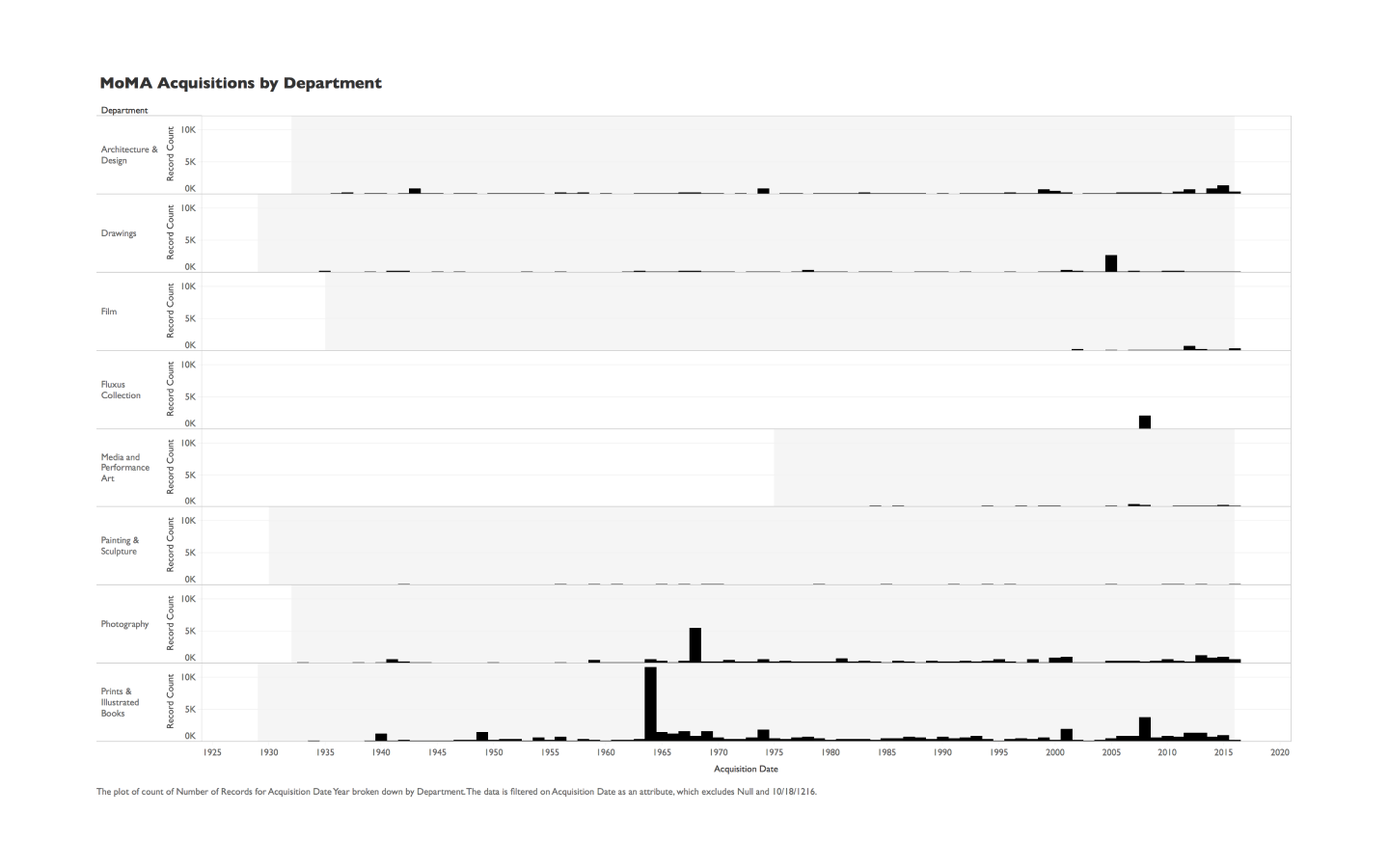
Pulling quantitative data from free text
It’d be interesting to compare the two time series, but I needed to get dates created formatted as years (numerical data) first.The data cleaning process was trial and error, and I spotted a few patterns with how dates were written. Spacing, punctuation, and formatting usage was surprisingly consistent, so these “rules” helped me create a script that splices and concatenates text.
Eventually, I was able to:
Fortunately, this is the Museum of Modern Art — there’s a limited date range, so checking for mistakes was easy.
- Get the year created for 97.5% of the records. For the remaining 2.5%, there wasn’t a known year or date range to begin with.
- Calculate the gap between dates acquired and created for 92.7% of records. The remaining 4.8% had only one of the two.
Fortunately, this is the Museum of Modern Art — there’s a limited date range, so checking for mistakes was easy.
Finding a story within the data
I then looked into the relationship between these variables:- The year something was created
- The year MoMA acquired it
- What kind of work it is, based on department. Medium and materials were so varied and specific that classification didn’t say much.
I then created a dashboard to present my findings to the class. Their comments helped me pick out interesting bits:
- Year created was more varied that expected. If MoMA acquired something 100 years old, is it still modern?
- There were a few acquisition spikes. What did the MoMA acquire in (very) large bulk? Where did it come from?
- When data was broken down by department, there were some drastic differences I wanted to call out.
View the interactive dashboard here.
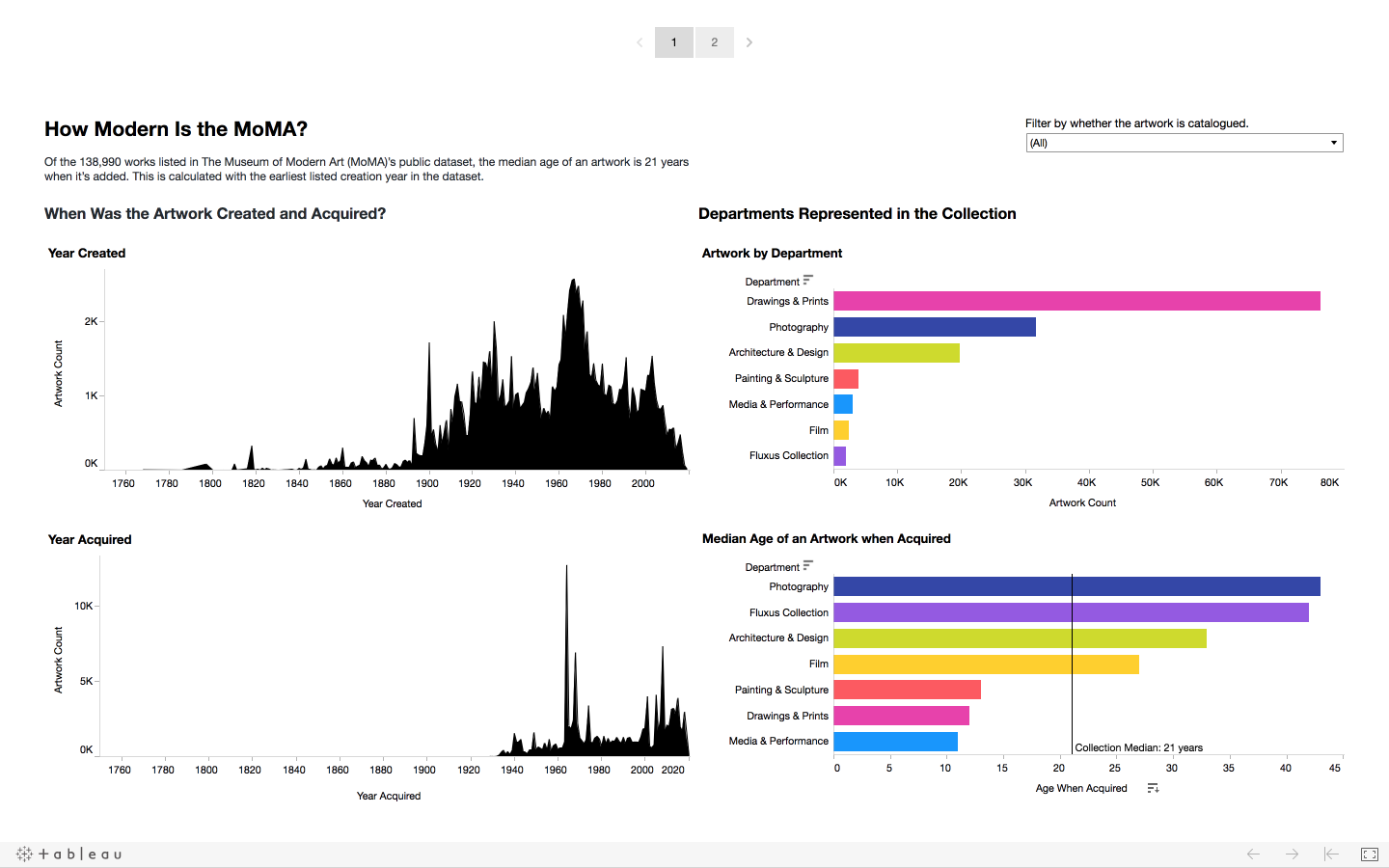
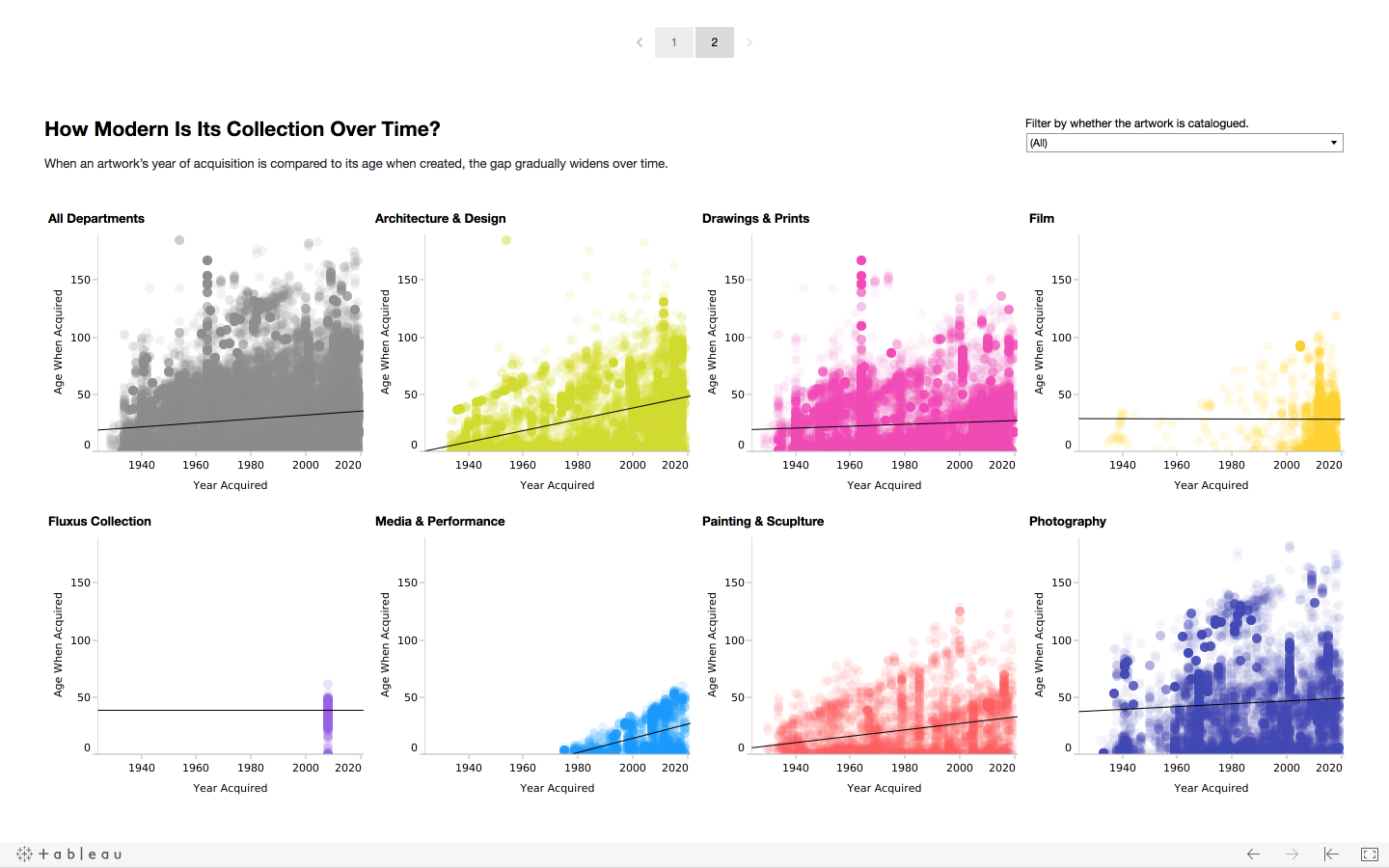
Putting the story together
Starting with sketching helped me figure out what kind of story to tell.- Write a general outline with a few questions to answer
- Draw some primary visualizations
- Pick a few ideas to develop into wireframes
- Add supporting visualizations and annotations
- Alternate between writing and visual design until it’s done.
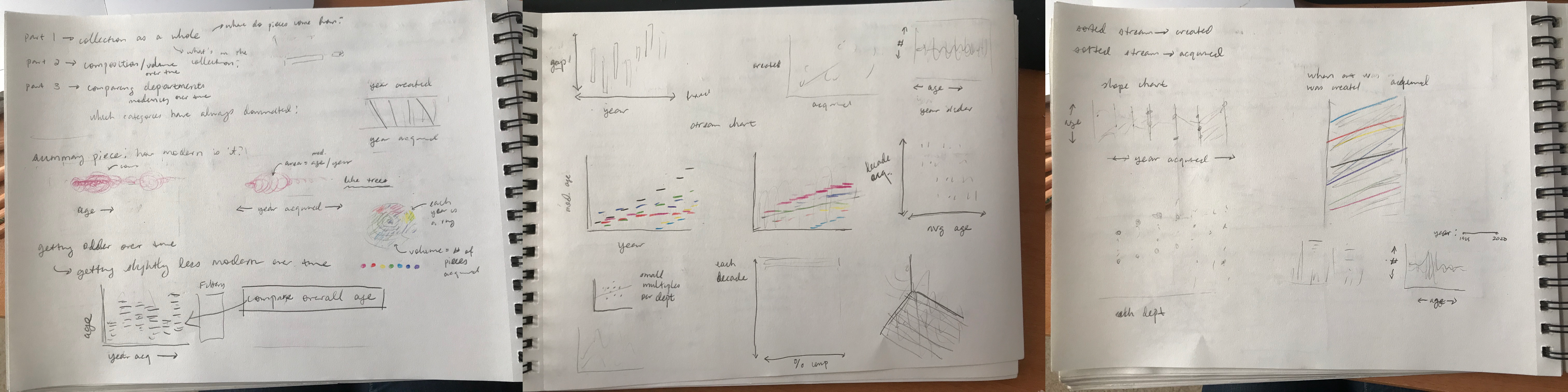
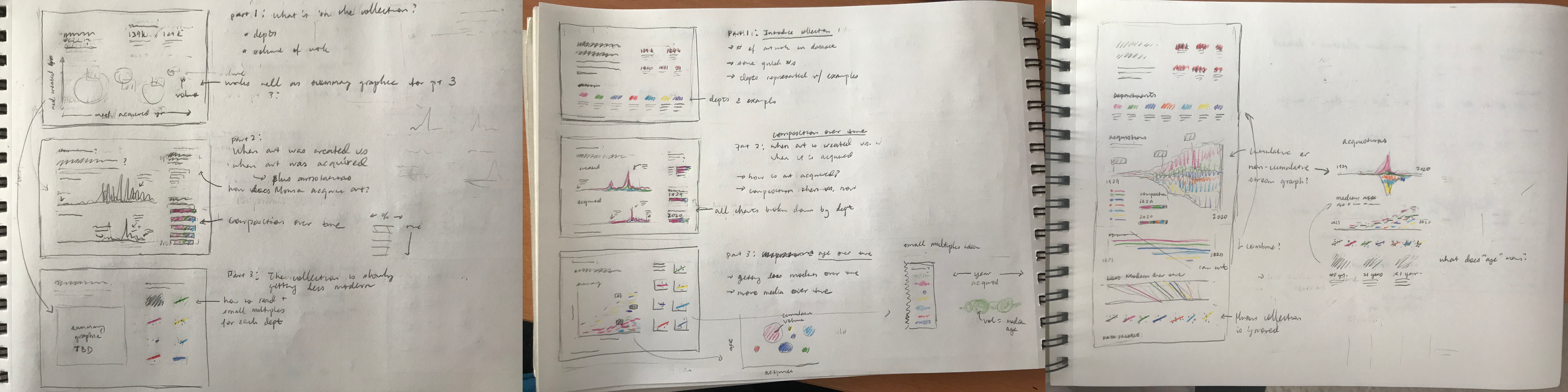
The final design
I worked some of the potential ideas above into the final narrative, either as their own sections or as interesting annotations:- Introduce MoMA, the data set, and the collection.
- What kind of art and design are in the collection? Which departments are represented?
- How did the collection change over time? Which departments came and went?
- How modern is the collection? How modern is each department?
View the project here.
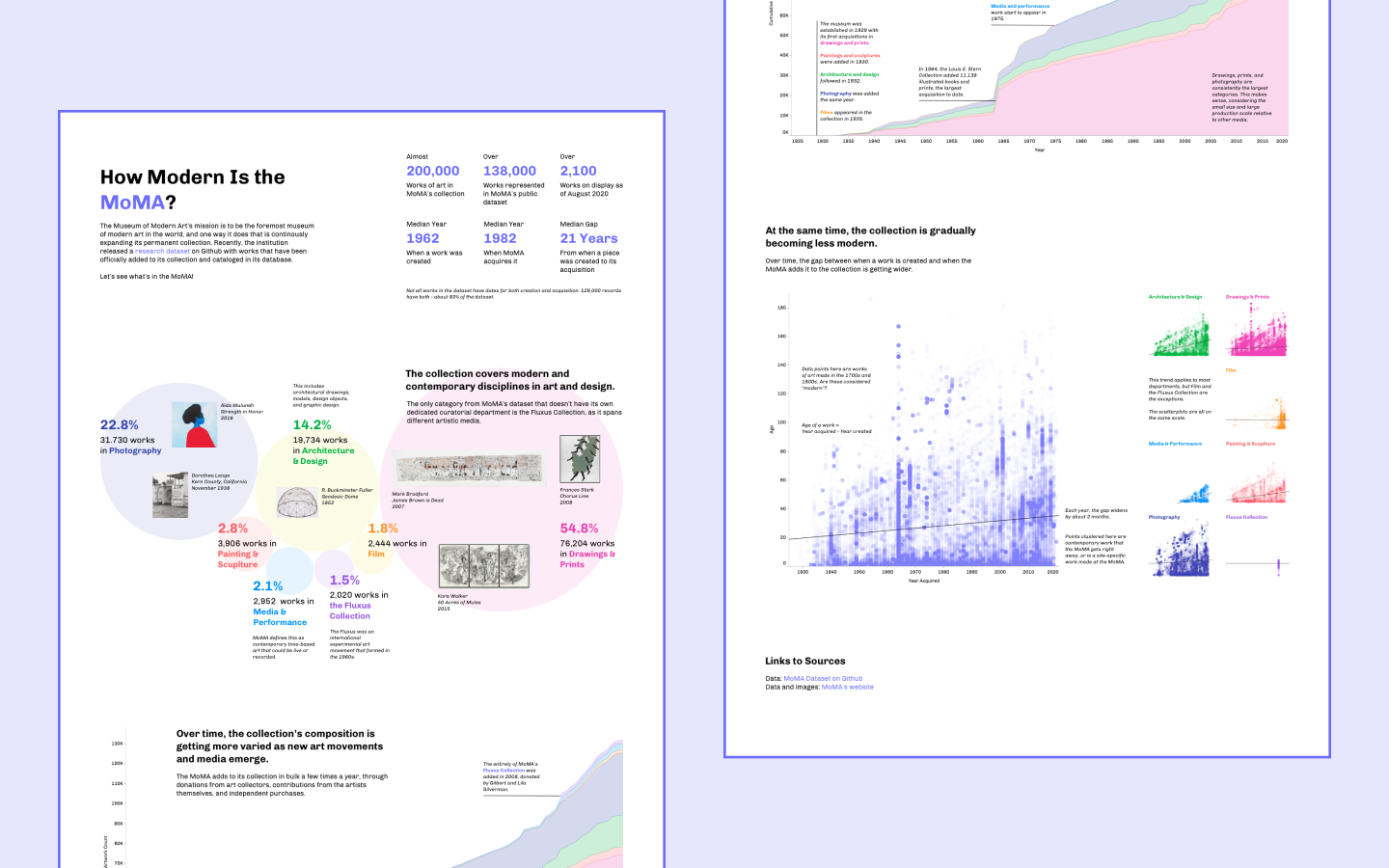